Self-Attention and Active Inference
COGNITIVE SCIENCE
Ajith Senthil
3/13/20231 min read
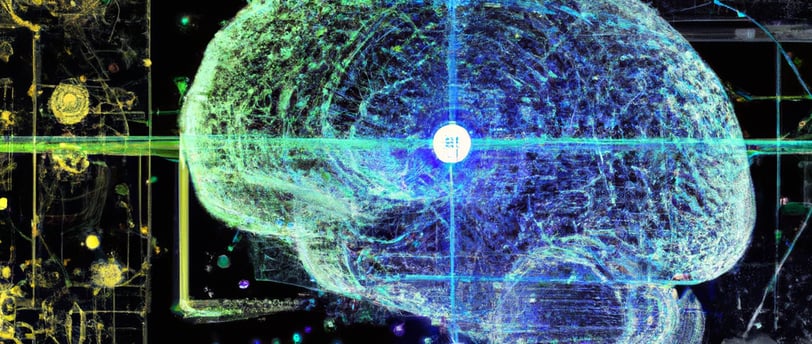
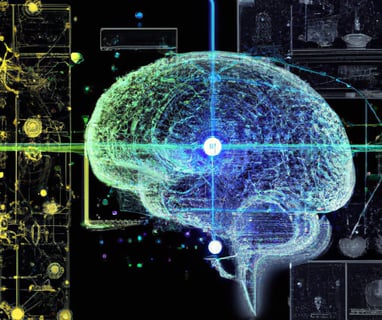
The search for models of cognition has led to the development of various systems that have become useful in different subdomains. These models rely on similar principles that connect many different models of learning. One such system is the Transformer architecture that uses self-attention mechanisms to take into account global context. These mechanisms are connected to word embeddings in semantic networks, which can use active inference and Bayesian inference to make many models of cognition seem like models of information metabolism.
The self-attention mechanism creates its own semantic network by training on a large amount of data that is structured by time. It extracts the relational dependencies between each word based on its position and context. The embeddings in word2vec, for example, do not take into account positional embeddings, so it may be a more homogenized semantic network than the one that would be in a transformer architecture trained on a large amount of text data.
In conclusion, understanding the connections between models of cognition and the insights gained from them can help us better understand the mind and behavior. The use of self-attention mechanisms in the Transformer architecture and word embeddings in semantic networks provide a powerful tool to extract relational dependencies between words and make predictions about future sensory inputs, using Bayesian inferential learning. These models are optimized by minimizing their perplexity and can be thought of as models of information metabolism.